Tabea Röber
UvA
Counterfactual Explanations for Interpretable Machine Learning: A Generic Framework
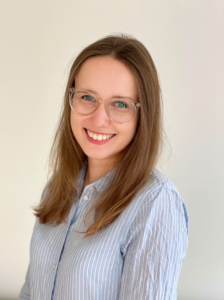
Counterfactual explanations are a popular post-how explanation method in interpretable machine learning. Several criteria that make a good counterfactual explanation have been put forward in the literature, and we propose an approach that addresses all of those in a combined framework. We demonstrate our results in a case study using credit risk data.